The convergence of AI, IP and web3
The murky world of copyright in generative AI may have a way forward
In my previous post on building brands, products and innovation processes with generative AI, I touched on the ethical implications of the technology. Central to that is how original works are scraped for large language models, how IP is attributed to creators and how they are therefore compensated. I referenced Packy McCormick’s hot take on a way forward, which could open up an entirely new dimension in how two of this decade’s most hyped innovations could come together:
AI will be web3’s ultimate best use case. People will need a way to own, permission, and benefit from their data as it feeds increasingly powerful models, and if open AI wins, decentralized ownership and governance of those models will be critically important. Looking back in a decade or so, I wouldn’t be surprised if we viewed all of web3’s early stumbles and mistakes as necessary experiments for the main event: governing AI in such a way that it doesn’t turn us all into paperclips, owning enough of it that we benefit from its use of our data, and rewarding open source contributors for their work.
This post will explore the intellectual property considerations for generative AI, including how original works and styles are used by learning models, the commercial realities this presents for both AI apps and brands that use them, plus the possible AI + web3 cocktail that could pave an equitable path forward.
Forgiveness, not permission
The startup ecosystem is no stranger to new technologies disrupting industries with a ‘ship it now, ask questions later’ approach. It has become a bit of a playbook now.
Step 1: Launch new tech in market
Step 2: Drive market demand for the innovation
Step 3: Wait for the industry backlash
Step 4: Achieve scale
Step 5: Wait for regulators to understand the tech, the industry then figure out a game plan
Step 6: Find a small compromise or regulatory workaround once beyond the point of return
Think about Uber or Lyft in ride-sharing, Airbnb in accommodation, Bird or Lime in micro-mobility. We’re currently living through an equivalent, permissionless phase for generative AI. As the tech and the industry continues to advance, the question of how to handle intellectual property considerations has become increasingly relevant. But this time, the incumbents getting disrupted aren’t corporations. They’re artists, writers and coders.
There are three main buckets of IP issues that this post will touch on:
Training data
Original or derivative works
Mimicking artist styles
These buckets are not necessarily discrete in nature, nor is this an exhaustive list of IP issues in AI. But these are some of the main points of consideration, especially when it comes to how AI generated work can be commercially copyrighted in the future.
Training data using copyrighted material
The reason for that comes down to how the learning models that power these AI apps use existing original works. Language models like GPT-3 are trained on large amounts of text, image and audio data from a variety of sources, including books, articles or websites (including Google, Reddit or Getty). This training data is fed into the model, which uses it to learn the patterns and structures of the language. Once the model has been trained, it can then generate content that is similar to the training data it has seen.
The training data that these language models use can be purposefully opaque. Take for instance OpenAI, which has an unreleased proprietary dataset, with hundreds of millions of images… much of which is suspected to be copyrighted. On the other hand, Stability AI’s Stable Diffusion uses a dataset of 2.3 billion, but this is completely open source.
Generative AI uses these datasets to create new works such as text, images, and audio. This presents a number of challenges when it comes to attributing ownership and compensating the creators of the original works that are used to train the AI models.
AI imagery: original or derivative?
This raises the question of whether AI generated content is original or derivative. An argument for the former is that the datasets are used to train the learning model, which fuel the AI with pattern recognition and parameters by which to recreate entirely new material. The output from the apps aren’t merely a ‘collage’ of the training data, but novel creations. Therefore attribution isn’t necessary and intellectual property rights should be attributed in full to the new work.
One counter argument to this is that, even if there’s no direct representation of the training data in the output content, it’s still derivative. I came across a wonderful comment by ‘Adpah’ on a Waxy article, which provided a strong case for this point of view:
It’s true, AI does not store the images. It analyzes them, derives patterns, rules, relationships etc., and stores its analysis results as abstract mathematical parameters.
It does not change the fact that you would not be able to build your tool without the data.
It is completely irrelevant that the data is discarded.
It is still a crucial component in your process of creating your software. Without it, it would literally not be possible.
This means, you are dependent on the data to build your tool.
But if you depend on the property of other people then you need to get their permission to use it for commercial purposes and reimburse them if they demand it.
Mimicking artist styles
Not only is this important for how an original work was used within a training dataset, but it’s important as it related to a specific artistic or design style. The Waxy article referenced above described a case study where an illustrator’s work was fed into Stable Diffusion’s learning model by a user (!), outside of the illustrator’s awareness or willingness. This resulted in the ability of Stable Diffusion (and its 10 million users) to exactly copy her illustration style.
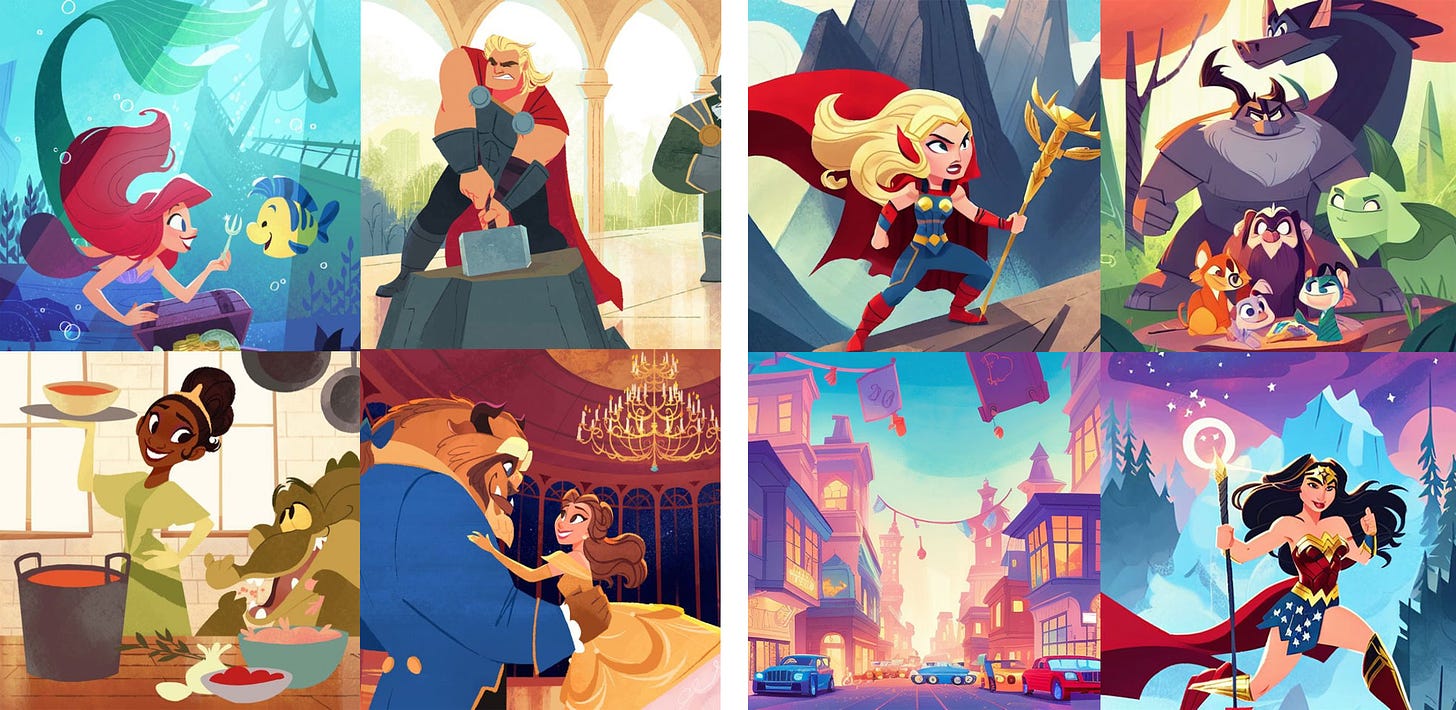
We’re entering Step 3 of the Playbook now (“wait for the industry backlash”) and beginning to see some of the commercial realities facing the applications, platforms and brands working in this space.
Current approaches to dealing with IP
Companies in the generative AI space are coping in different ways. Some are leaning in, some are protecting themselves, some are problem solving. Here are a few approaches we’re seeing in the world:
Approach option 1: Get sued.
Microsoft’s Github and OpenAI are mired in a sizable class action lawsuit over their Copilot app (essentially autocomplete for code), alleging copyright infringement as it reproduces open-source code and “relies on software piracy at an unprecedented scale”.
Approach option 2: Be an ostrich.
Getty has banned AI-generated content appearing on it’s platform, citing fears of future copyright claims. With images like the below being churned out of Dall-e, Midjourney and Stable Diffusion, their reasoning can be understood.
Approach option 3: Partner.
A very different approach in stock libraries comes from Shutterstock, who have entered a strategic partnership with OpenAI. Shutterstock have licensed their own library of images to OpenAI and are working on solutions to fairly compensate artists whose work trained dall-e. In addition, they are developing best practices to fairly compensate artists whose work is drawn from to create generative images prompted on Shutterstock’s new tool.
Approach option 4: Innovate.
Adobe is forging a slightly different model, where it leverages its own stock library to power its AI engine, Adobe Sensei. Sensei is not a standalone app, but is integrated across Adobe’s suite of products where it is leveraged to create more efficient workflows and creative tool options. This walled garden allows for control about the training data used for its learning model and certainty about the ability for commercial usage of any content that is created from using their platform.
In addition, Adobe is also making strides in content provenance with the Content Authenticity Initiative. This has a focus on providing proper authentication of what data is used (and where) and tracking changes. Adobe also announced partnerships with Leica and Nikon, who are building content authenticity into camera capture. This ensures that creators can attach themselves to their intellectual property at the time of capture for accurate attribution down the line.
The convergence: AI x web3
In my opinion, the provenance approach is where the industry should focus. It has challenges, for sure, notably those of complexity and compensation. Keeping track of the metadata, identifying where and how an asset is used either directly or indirectly in AI generated content and enabling content originators to see how their content is being used and to be compensated sufficiently when their work is referenced.
This is where web3 could provide a hugely valuable service to the industry.
Some questions that it should seek to address:
If an original work was used to train a learning model and a text-to-image prompt referenced the work, can creators have visibility into that derivative work?
Can the application layer track when a piece of content is directly referenced in derivative content?
Can the application layer track when a piece of content is used to create parameters in derivative content?
Can the marketplace determine a fair compensation to the original creator relating to how much influence the creator’s work has had on a derivative piece of content?
Can the creator engineer a smart contract into its original work NFT outlining licensing terms?
Can the marketplace automate and execute licensing terms and payment based on the pre-defined smart contracts?
This isn’t a silver bullet, nor have I looked into the technical feasibility of this sort of approach. But it appears that a sensible solution is an opt-in model with pre-defined licensing and payment terms built into smart contracts that can be automated, executed and enforced instantaneously and at scale.